Greater efficiency through machine learning with Gewobag
- Energy & Utilities
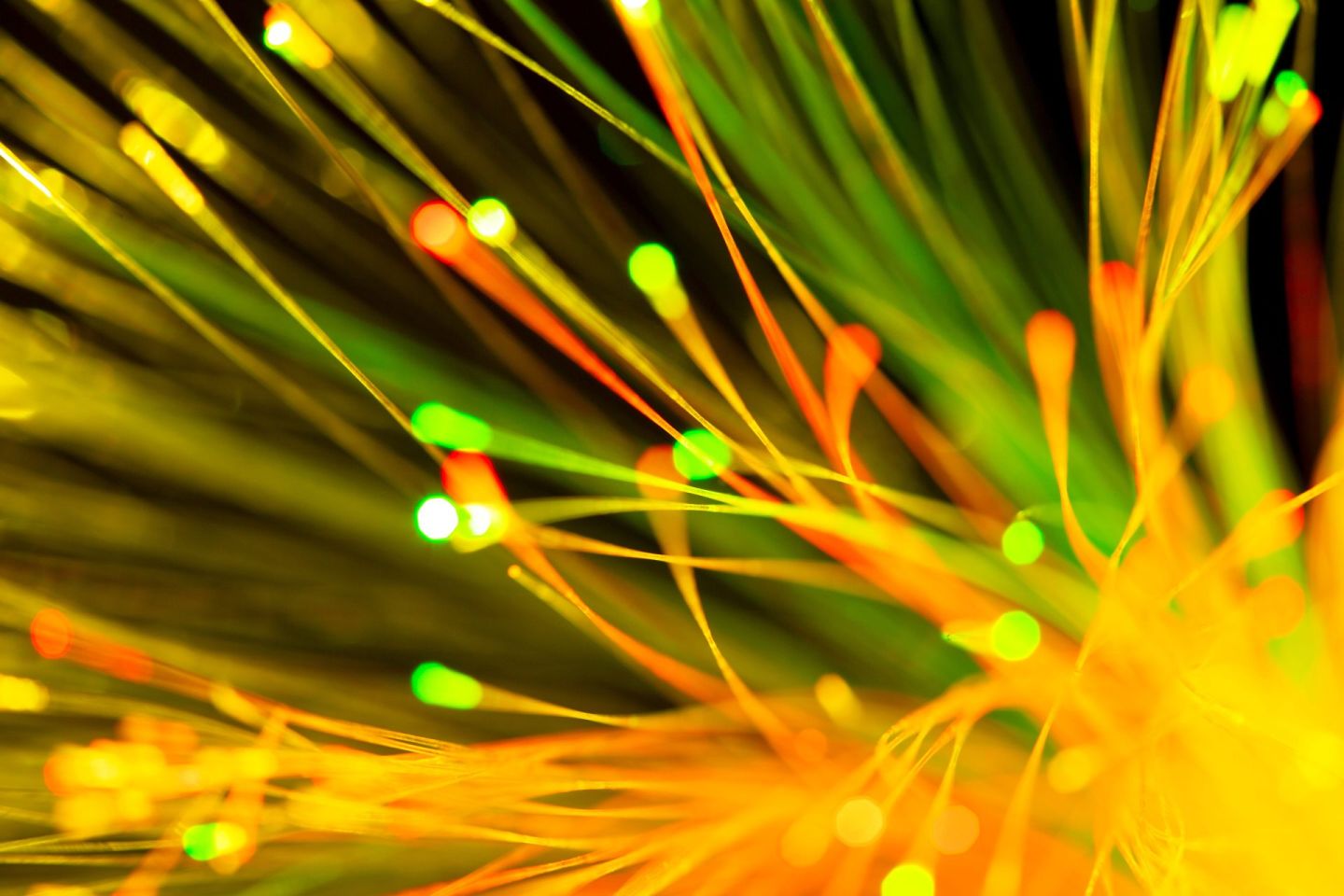
Gewobag is a housing company based in Berlin and one of the largest property companies in Germany. For over 100 years, the company has been pursuing the goal of creating affordable housing in the capital. Successfully: Like the city of Berlin itself, Gewobag experienced vigorous growth in the past few decades. The company now has a portfolio of nearly 80,000 apartments. By 2026, the number of apartments is expected to increase steadily to 86,500 units through new construction, acquisition and modernization of existing buildings.
Meanwhile, Gewobag is much more than just a property company. As a municipal company, it plays an active role in society. Social, economic and ecological sustainability thus form the three pillars of its actions. For example, as a founding member of the Housing 2050 initiative, the company has committed to the goal of making all of its building stock climate-neutral by 2050.
To achieve this ambitious goal, Gewobag focuses on the digitalization of the housing industry. With the help of more efficient processes and a better database, resources can be spared, emissions reduced and workflows organized more efficiently.
Smart buildings and data-based decisions – the future of the property industry
As in many other sectors, there is an increasing trend towards digitalization in the real estate industry. Buildings are evolving into smart buildings. The use and review of data is playing an increasingly important role. For example, inefficient operating patterns can now be identified, optimization potential derived, faults and problems can be detected at an early stage, thus enabling operating processes to be optimized. In addition, building data forms the basis for sustainable decisions in the property sector as well as for meeting legal requirements (e.g. ESG reporting).
Thus, the use of energy data portals in which high-quality, complete and plausible data is collected and assessed is becoming increasingly important. The use of AI technologies and machine learning can help to reduce manual effort and make processes more efficient in the long term. Gewobag Energie und Dienstleistungsgesellschaft GmbH, Gewobag’s in-house energy service provider, has implemented this approach in collaboration with Wavestone subsidiary qdive. The aim was to implement an automatic plausibility check of heat consumption data.
With data-driven operating processes, we will experience a new dimension of efficiency and sustainability on the way to smart buildings.
Automatic plausibility check of consumption data through machine learning
As part of the preparation of the annual consumption billing for several hundred properties, the plausibility check of the individual consumption quantities recorded by heat meters was conducted in advance. This process involved a considerable amount of work, as the data required for the analysis had to be extracted from various internal and external data sources and processed and assessed in Excel. Implausible heat consumption often required an on-site inspection and, in many cases, the replacement of defective meters. This led to further billing delays.
In order to optimize the process, machine learning was used for the annual validation of heat quantities. Machine learning is an area of artificial intelligence that enables computers to learn from data, recognize patterns and make predictions without explicit programming.
Through the manual plausibility check of heat consumption in recent years, we know which constellations are plausible and which are not. This data can be used to train a machine learning model that can automatically classify new data into “plausible” and “implausible”.
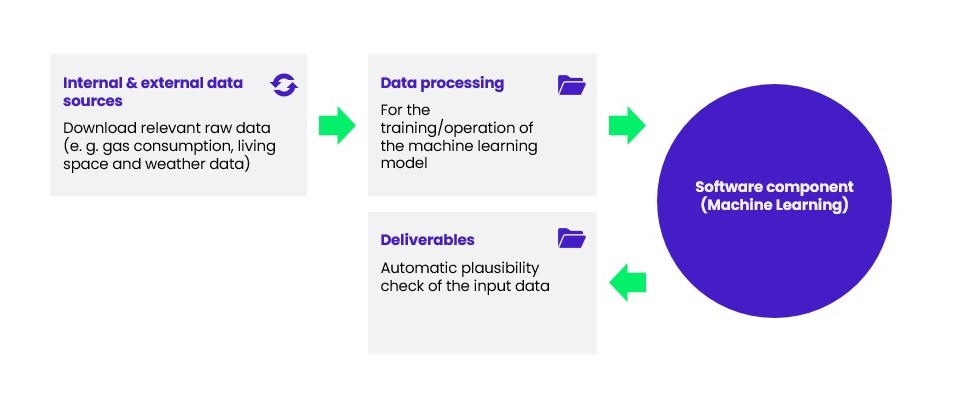
Explanation of results to focus on automated validation
When automating the plausibility check consumption data, a special focus is placed on the explanation of the results. In order to understand the automatically generated plausibility check assessments, the basis for the decision is displayed for each individual result based on the incoming characteristics, e.g. efficiency or specific consumption.
The model then consistently implements these requirements. It automatically flags implausible entries almost in real time, which previously had to be identified manually in a time-consuming process. The very high level of accuracy of the results produced are reliable and can be used productively. Meanwhile, it provides a transparent base for valid classification.
In the future, regular plausibility checks will be carried out during the year. Internal and external data sources will be connected to the machine learning model via API for this purpose. This means that regular checks or checks as required can also be carried out automatically outside the accounting period without a great deal of additional effort. For example, defective meters can be detected and replaced at an early stage.
By using the machine learning model, our manual effort in checking the validation of consumption data has been reduced to a minimum. This frees up resources for other tasks.
Digitalization of the housing sector: The potential of smart technologies
The validation check illustrated that uses machine learning can enable the automation of time- and cost-intensive processes and thus directly reduces administrative costs. However, it is only a first step on the way to digitalizing the housing sector.
AI and machine learning can make a decisive contribution to economical and ecological sustainable management of property portfolios in the future: By analyzing weather forecasts and residents’ usage patterns, for example, energy consumption can be optimized, thereby reducing costs and CO2 emissions. There is also potential in the area of maintenance and servicing that can be exploited with intelligent solutions. Continuous and automated monitoring of the building technology and intelligent forecasting make it possible to identify problems at an early stage and efficiently plan maintenance measures before major damage occurs. This minimizes downtimes and reduces repair costs.
Consequently, technologies such as AI and ML have the potential to permanently change the property sector in every respect and create real added value for society.
Author
-
Daniel Böhm
Senior Manager – Germany, Munich
Wavestone
LinkedIn