2025 AI Cyber Benchmark
Published March 31, 2025
- Cybersecurity
- Data & AI
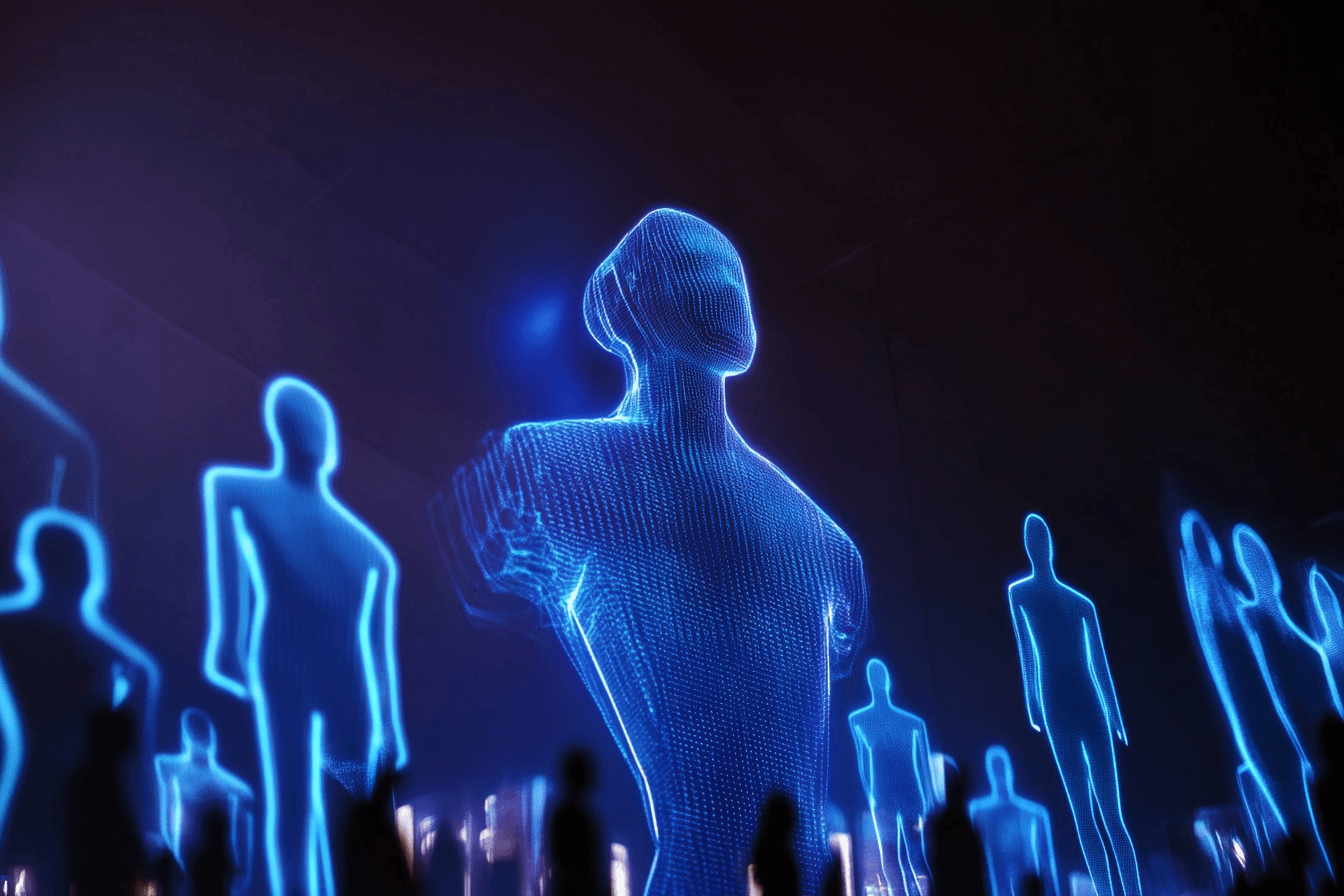
Are large organizations ready to handle AI security risks?
Over the past two years, since the public release of efficient generative AI systems, large organizations have accelerated their adoption of artificial intelligence, developing promising use cases to support their business operations. However, AI systems—which differ from traditional IT applications in several ways (non-deterministic, non-explainable, utilizing a wide variety of data, etc.)—introduce new security risks. Organizations must therefore adapt their governance, methodologies, and tools to align with this new reality.
In this context, to help organizations identify their priorities and areas of focus, as well as to gain insight into market maturity, Wavestone has developed its AI Cyber Benchmark. This initiative assesses an organization’s maturity regarding its AI security practices based on the five pillars of the NIST Cybersecurity Framework: Govern, Identify, Protect, Detect and Respond.
This assessment is intended for all organizations that have adopted AI use cases, ranging from simple users leveraging public AI tools or SaaS solutions, to those developing proprietary models and offering them to their clients.
These organizations utilize AI functionalities embedded in the software they already use. Their primary risks are related to data security and third-party provider selection. Nearly all companies are concerned by this usage, whether through “shadow AI” or the planned integration of new AI features and must ensure appropriate governance frameworks are in place.
These organizations design AI systems internally, with data scientists building upon existing frameworks or pre-trained models. Their main risks lie in the surrounding infrastructure and dependencies of the AI models. A robust methodology is required to properly identify and mitigate risks at the system level.
These organizations develop proprietary AI models, often with the intent to commercialize them. They face a broader spectrum of AI-related risks, from model design to deployment and client usage.
Each of these approaches carries distinct levels of risk and requires tailored mitigation strategies. After conducting an initial wave of assessments with 20+ organizations, we present here the results and key insights gained, combined with our experience from AI security engagements carried out over the past two years.
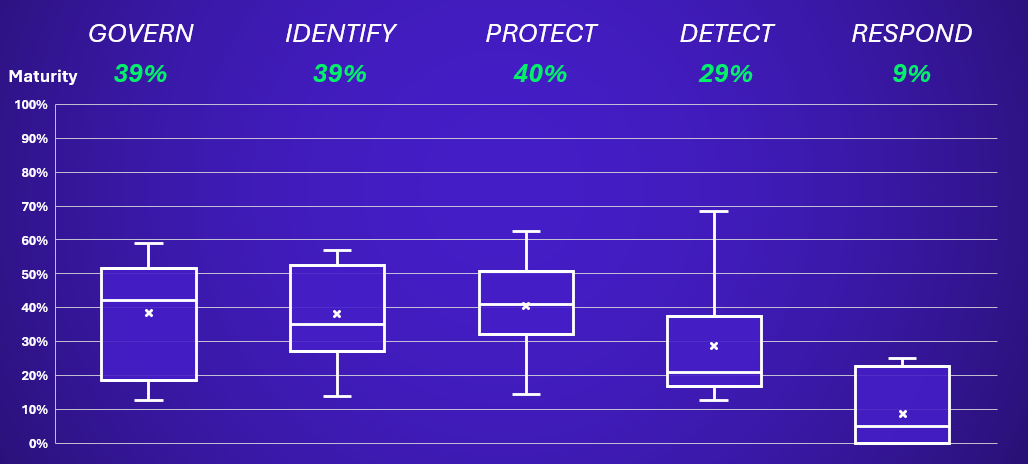
Key takeaways from our AI Cyber Benchmark
- The risks of GenAI are multiple (cybersecurity, data protection, bias, transparency, performance) and highly specific.
- New AI technologies introduce three novel attack types: poisoning, oracle, and evasion attacks.
- In response, international regulations are multiplying, adding complexity for multinational companies.
- Security actions vary depending on AI use cases, categorized into three types: users, orchestrators, and advanced creators.
- While 87% of companies in our panel have defined governance elements, only 7% have the necessary expertise to address emerging risks.
- The most mature companies tackle the skills challenge by adopting an integrated governance model.
- 64% of companies have implemented an AI security policy to establish a baseline level of security for AI projects.
- 72% have adapted their security processes in projects to address AI-specific risks.
- 40% of companies have adjusted their third-party maturity assessment processes for AI-powered solutions.
- 43% of companies have incorporated security criteria to guide model selection for use cases.
- Only 7% of companies are properly equipped (in-house or with vendor support) to defend against model-specific attacks.
- To validate trust levels, AI red teams remain the preferred approach. Only 7% of companies have the in-house expertise to conduct them and must rely on external specialists.
- AI applications are rarely monitored via the SOC. However, infrastructure and application logs are generally well collected (71%) to support investigations in case of incidents. AI model forensics remains highly complex technically, with only a few organizations worldwide capable of performing it.
AI impacts cybersecurity in two other ways: it strengthens defense by automating certain tasks, but it is also exploited by cybercriminals, with an increase in deepfakes, AI-powered phishing, and AI-co-written malware in 2024. Organizations must incorporate these threats into their monitoring and adopt AI tools to counter these new attacks. You can find more information here: The industrialization of AI by cybercriminals.
Our feedback from the field
What are the trends we identified when it comes to securing AI?
The market responded quickly to the arrival of AI. Today, 87% of the organizations in the benchmark have defined a governance to tackle the topic of Trustworthy AI. Indeed, cybersecurity is just one leg of AI Trustworthy, aside privacy, bias management, robustness, accuracy…
Mainly, we observed two approaches:
- An integrated model (around 60% of our clients), via an “AI Hub” bringing together key skills around AI (legal, security, privacy, CSR) to facilitate communication and quick adoption of topics. This helps centralize use cases assessment, building an AI trustworthy community, and accelerate teams’ upskilling.
- A decentralized model (around 10% of our clients), where AI specificities are tackled via existing teams and processes. Although slower to implement, it helps creating a dedicated governance structure and prevent redundancy with existing processes.
But then, how to effectively manage AI risks in practice?
71% of the organizations in our benchmark have adapted their project lifecycle process to the risks of AI. 64% have defined an AI security framework, either by establishing a dedicated security policy or by adapting their overall security corpus. And this should be the first step for any company trying to set up an AI security framework.
One key success factor is to not reinvent what already exists and build on existing processes and documents, adding where relevant risks, measures, controls, and verification steps specific to AI. For instance, the risk qualification questionnaire can include specific questions on AI, such as:
- What is the context and intended purpose of this AI?
- Would the output be utilized by a human or directly as an input in an automatized process?
- What is the expected level of performance of the AI system?
- What would be the impact of a malfunction on individuals
However, the issue of skills remains a key challenge: AI is a specialized topic, and expertise can be scarce. Only 57% of the organizations in our panel have identified internal or external experts on AI security topics. Therefore, training, awareness, and identifying missing expertise to adapt these recruitment strategies will be essential.
Many classic security measures can be easily adapted to artificial intelligence, but this is not enough, especially for critical use cases. The level of security required depends on your AI posture, as described in the introduction of this study:
- AI users: Primarily leveraging third-party AI solutions, organizations in this category must focus on data security and supplier risk management. 40% of organizations in our benchmark have adapted their third-party evaluation methodology to AI providers. Key areas of attention include AI risk awareness, governance, policies & compliance (e.g., AI Act and to avoid “Shadow AI”), and the implementation of a third-party AI risk framework.
- AI orchestrators: These organizations integrate third-party AI models into their products/services. Security priorities include ensuring the robustness of AI platforms and securing data repositories. 43% of organizations have already implemented a model selection process that includes security criteria. It is crucial to secure AI platforms, protect AI-accessible data repositories (e.g., RAG-based architectures), and enrich security tooling for critical use cases. As AI integration grows, implementing MLSecOps practices becomes essential.
- AI advanced creators: Organizations that develop proprietary AI models bear full responsibility for their security architecture and require a high level of maturity. As of today, only 7% of organizations in our panel have implemented measures to detect and protect against malicious prompts and other AI threats. Priorities include securing all tools and processes used to build AI by MLOps teams, ensuring advanced protection for ML key assets, and enhancing detection and response capabilities.
Each of these AI adoption postures comes with its own set of risks and necessary mitigation strategies. Fortunately, the market for security solutions is becoming increasingly diverse, including with open-source solutions. Organizations must ensure that they do not solely rely on the native security measures of their vendors or on their assumptions about cybersecurity and instead implement a level of protection tailored to their risks.
Monitoring and testing are crucial to complement protection measures. While many tools are already available, organizations often have not yet defined their strategy in this area:
- Regarding monitoring, 71% collect application logs, but only 13% send them to their SOC to generate alerts. Organizations are generally more mature in the continuous evaluation of their model’s performance, with 36% of organizations having defined metrics in this area, especially those with established data science processes.
- Regarding AI pentesting (also named AI Red teaming), 64% of organizations have a specific testing process in place for their use cases, but typically only for basic attacks and manually executed. Only 7% have implemented a testing process that includes automation and conducts advanced tests tailored to the algorithms and technologies used.
For organizations, it has become essential to define their strategy on these two aspects: which are the most critical use cases that must be monitored and tested? How can I train my SOC teams to effectively handle alerts? How can I scale up to test use cases that are rapidly evolving?
The area of AI incident response remains uncharted territory: maturity on this subject stands at 9%. Many organizations still rely on their general incident response and investigation processes, without including AI-specific aspects. Only 29% have started to incorporate incident response scenarios involving AI systems.
As critical use cases begin to emerge, particularly with the arrival of agent-based AI, it will be necessary to adapt response capabilities. Initial initiatives have already emerged: Carnegie Mellon University has established an AI-CSIRT to analyze and respond to AI incidents, while ANSSI, the French Cybersecurity Agency, conducted a large-scale crisis exercise on an AI-related incident scenario during the last AI Summit.
Securing AI should be a priority, but let’s not forget that is just one of the facets of AI’s impact on cybersecurity. AI can help strengthen your cybersecurity: concrete use cases are emerging to automate and accelerate certain tasks. From multilingual awareness facilitation to preprocessing security alerts, and even pre-analyzing security questionnaires from your suppliers, there are already avenues to explore to assist your teams. We have witnessed real field feedback showing an increase in efficiency ranging from 15% to 30% depending on the use-cases.
Authors
-
Gérôme Billois
Partner – France, Paris
Wavestone
LinkedIn -
Thomas Argheria