Selecting the Right AI Use Cases: The Key to a Winning AI Strategy
Published November 20, 2024
- Data & AI
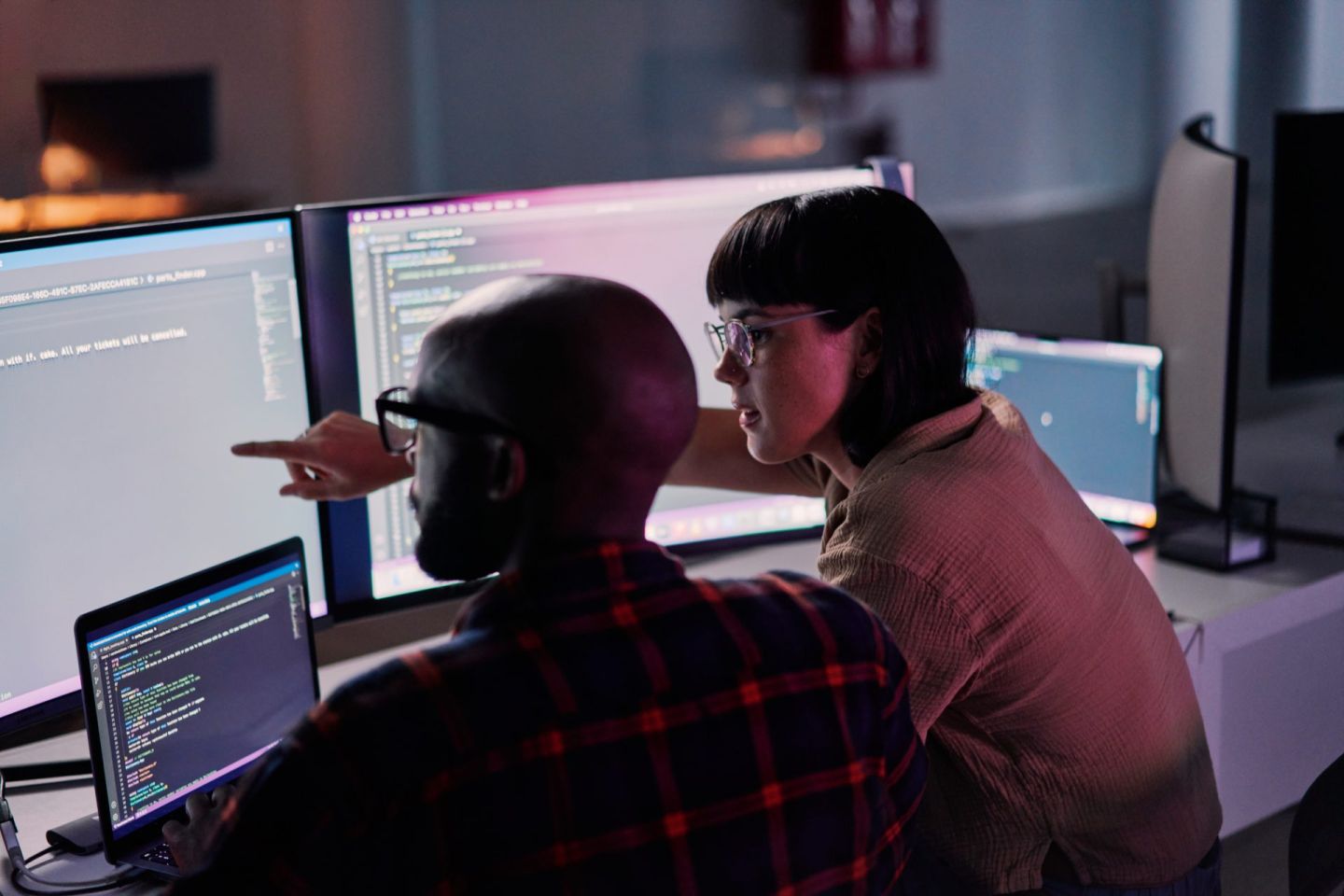
Successfully adopting new technology, like AI, hinges on the success of the first use cases. When these initial projects deliver real business value and meaningful benefits to stakeholders, adoption accelerates. But when they fail to address key challenges or fall short of expectations, disappointment takes over, dampening the organization’s enthusiasm and reducing the chances of long-term success. To avoid this pitfall, it’s essential to make use case selection a core focus when introducing AI into the business.
Aligning use cases with strategic objectives
Assessing the potential business value of use cases and monitoring industry trends is important, but relying solely on these can be misleading. Overemphasis on what others are doing may distort the view, leading to incorrect conclusions. Understanding how technology impacts the market, business model, and organization is crucial. Evaluating use cases from different perspectives provides insights into benefits, risks, and requirements, helping to shape and update strategies and make informed decisions – and removes blind spots.
Set the stage
The organization’s strategic goals provide the necessary context for selecting use cases. Often, new technologies require an exploratory approach that aligns with broader innovation goals. Over time, use cases should reconnect with strategic goals and the organization’s vision.
Understanding the organization’s strengths and technology readiness is essential. Applying maturity models from sources like Gartner or Microsoft can help set the stage. Organizations at early maturity stages, where learning is key, should approach this differently than those at advanced stages.
It’s important to manage expectations and set a baseline about what technology can and cannot do. In the hype surrounding technologies like Generative AI, possibilities may seem limitless, leading to unrealistic expectations. Unchecked, this can result in the selection of doomed use cases. Conversely, too much skepticism can lower the bar, leading to underwhelming outcomes. Regularly updating the baseline of evaluation criteria can mitigate these risks.
Stick to a structured process
Introducing new technology in an organization is an innovation process. A structured approach fosters creativity while minimizing surprises by identifying risks early on. Incorporating elements of lean experiments and the stage-gate model allows for rapid experimentation with iterative feedback loops, while also adding quality gates to evaluate progress.
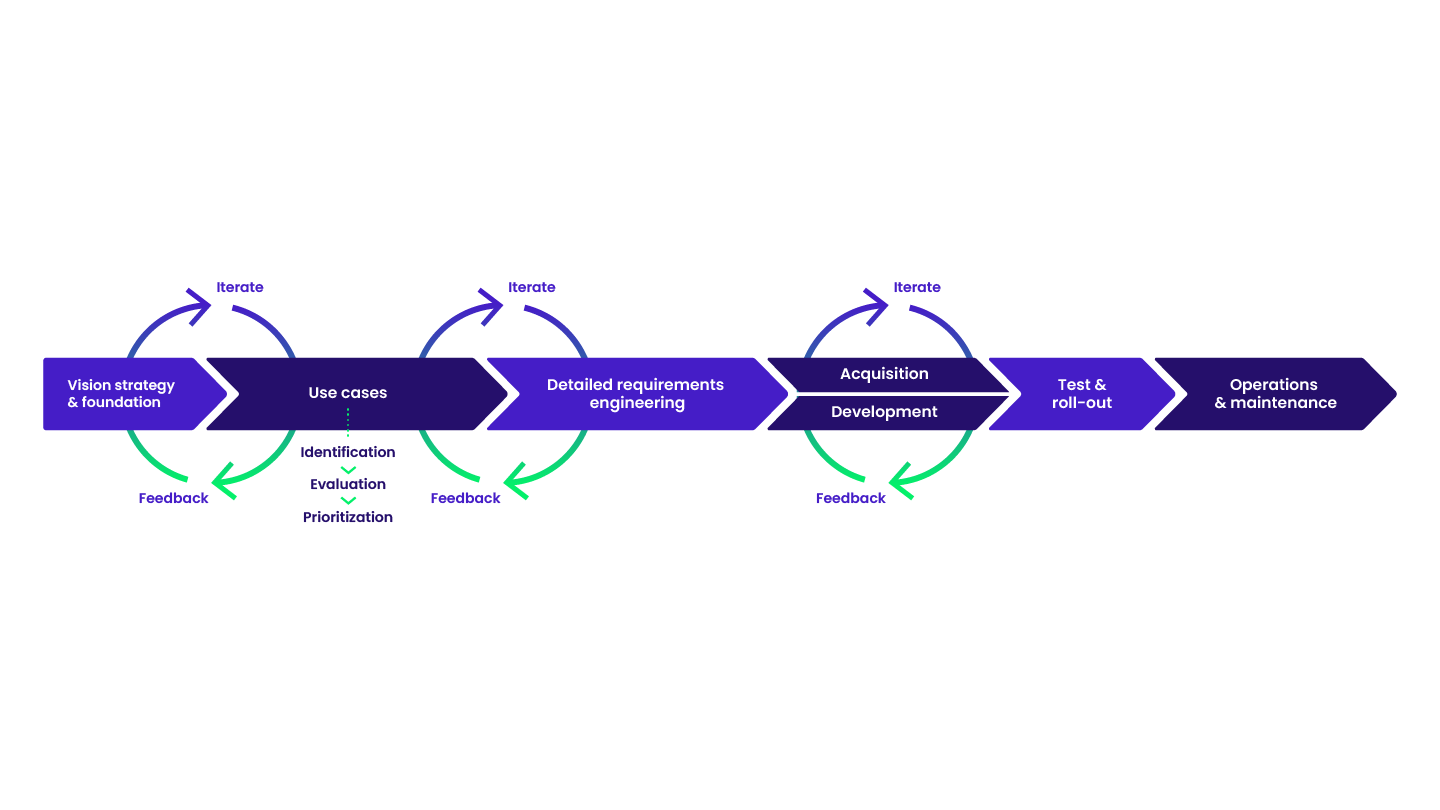
Step 1: Use case identification
Objective: Create an unevaluated list of business problems that could theoretically be solved with AI
The so-called law of the instrument often leads to the tendency of using new technologies to solve problems before they are fully validated, and before it is clear whether the technology is truly suitable or effective, as Warren Buffet illustrated with the saying, ‘…to a man with a hammer, everything looks like a nail.’ The “Use Case Identification” step ensures that the right business problems are identified and clarifies who faces these challenges. Begin by discussing current and future business issues, then explore how new technologies can address them.
Step 2: Use case evaluation
Objective: Develop a shared understanding of the value and complexity of the unscored use cases and create a scored list of use cases.
To assess business value, it’s crucial to understand not just what value a use case creates, but how it creates it. Value may manifest as increased productivity, improved quality, enhanced insights, or better customer experiences. The importance of these benefits varies by organization, depending on how well they align with strategic goals like growth, profitability, market position, or innovation. For instance, a growth-focused company might prioritize use cases that enhance customer experience over those that primarily boost productivity.
Complexity, on the other hand, encompasses factors such as effort, cost, duration, and risk involved in implementation. These must be weighed against the organization’s existing IT infrastructure, data capabilities, and skills. In the rapidly evolving AI landscape, compliance, ethics, and regulatory requirements further complicate matters – such as the AI Act. For example, stricter regulations might add red tape, significantly reducing the potential business value of certain use cases.
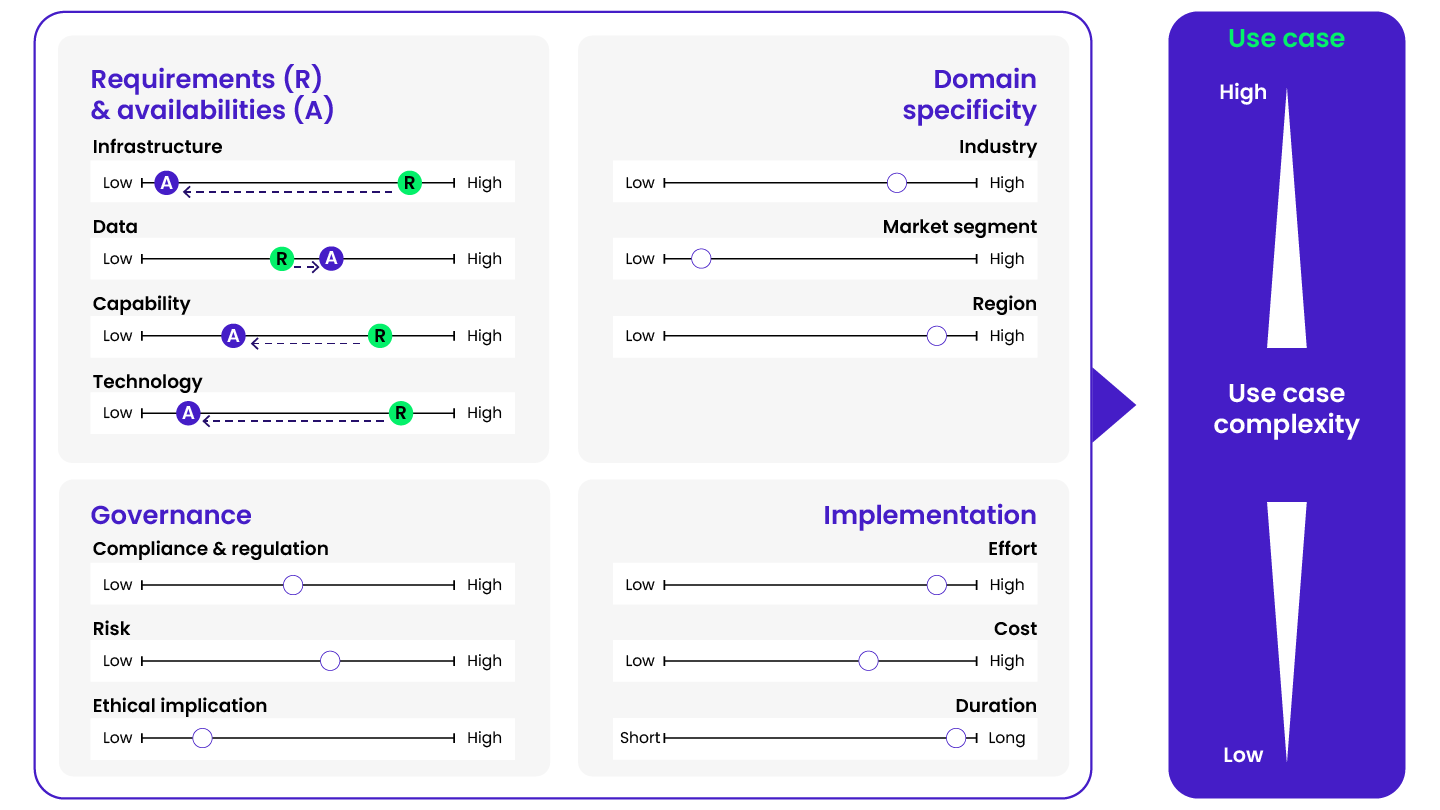
Step 3: Use case prioritization
Objective: Prioritize use cases and select those for testing or implementation.
Organizations should select use cases that deliver meaningful business value and align with strategic goals, considering their technological readiness. Newer AI adopters might focus on low-complexity, moderate-value use cases to better understand the benefits and challenges of AI. In contrast, more experienced organizations may target highly complex use cases with transformational potential.
Using a prioritization matrix can help visualize, support, and document the selection process. Introducing a quality gate at the end of this process enhances transparency, provides additional feedback, and secures management and leadership buy-in for the next steps.
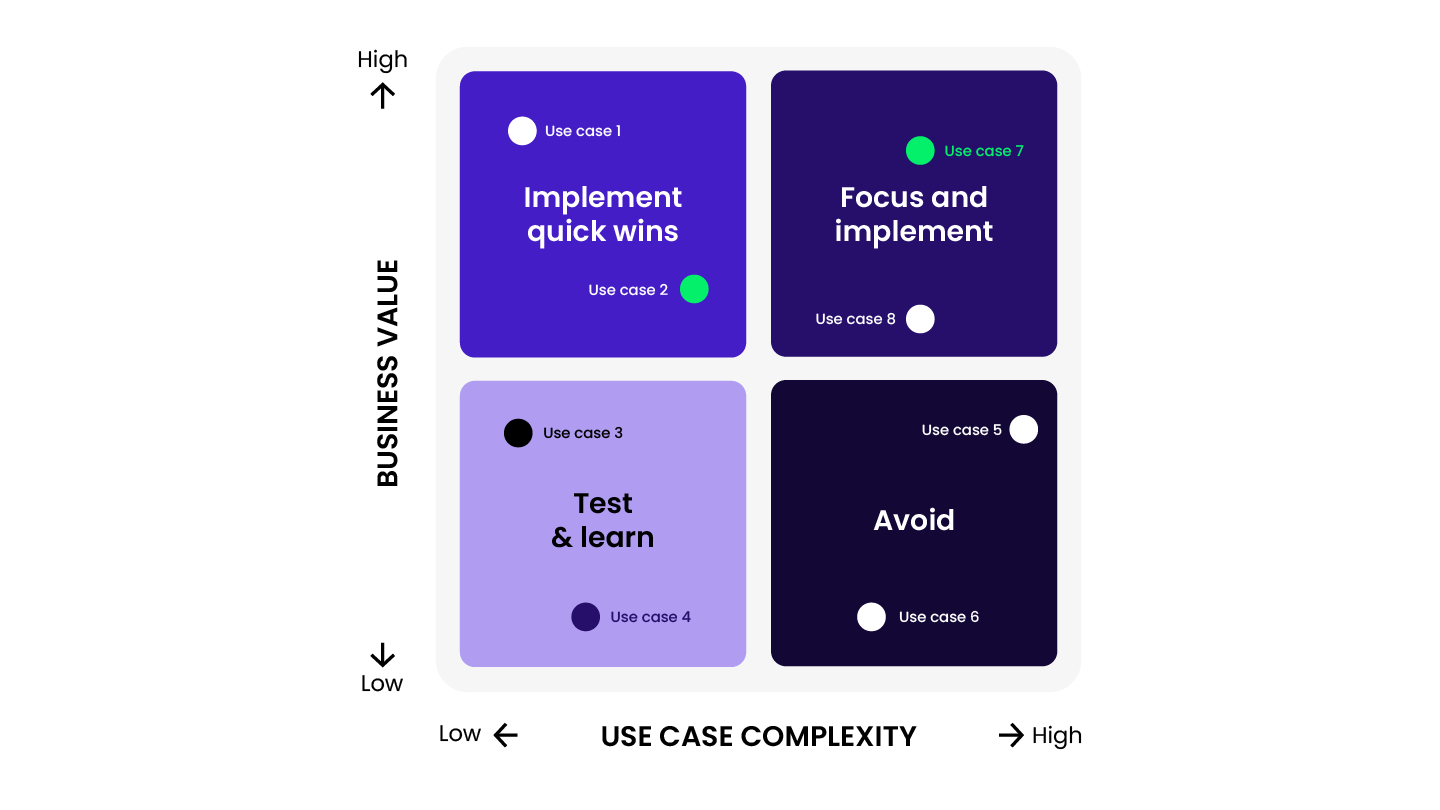
Mobilizing collective intelligence
The use case selection process demands diverse skills for reliable outcomes. Technical expertise is crucial for assessing feasibility, while subject matter experts offer domain-specific insights into business value. Risk and compliance, often overlooked, are key in evaluating complexity, especially with rapidly evolving technologies like AI. Involving other business experts, such as those in sales and marketing, is essential for understanding market potential. Although they may not be part of the core evaluation team, their feedback at key stages is invaluable. Collaborative reflection not only uncovers blind spots but also fosters shared conviction, driving the adoption of selected use cases.
Identify, evaluate, select — and repeat!
Use case selection should not be a one-time activity for a new technology, but rather an ongoing, iterative process. In a dynamic environment, parameters such as technology, regulations, and competitive strategies can change rapidly. A solid use case may quickly face new challenges or become obsolete as better alternatives emerge. To stay ahead, shift focus from immediate concerns to a broader perspective through scenario planning and trend monitoring, which helps identify risks early.
However, excitement over a new technology and the push for rapid execution after a use case is approved can lead to tunnel vision. This may cause missed learning opportunities or projects that underperform, ultimately hindering technology adoption. A structured, recurring process allows organizations to monitor key indicators, spot early warning signs, and promote continuous learning. This approach enhances risk management, improves project planning, and sets realistic expectations for time and revenue. Ultimately, it enables more agile adjustments to strategies as new insights emerge.
Building a dynamic approach
Selecting use cases for new technology is a dynamic process that requires ongoing monitoring, evaluation, and adaptation. A structured and iterative approach helps organizations avoid tunnel vision, sunk cost bias, and unrealistic expectations. It also fosters continuous learning, data-driven decision-making, and scenario planning, ultimately increasing the likelihood of successfully adopting and implementing technologies that align with strategic goals and meet customer needs.
Finding success with AI
In the ever-evolving landscape of AI, selecting the right use cases is not a one-time decision but an ongoing process that demands regular evaluation and adaptation. By maintaining a structured, iterative approach, organizations can stay agile, maximize AI’s potential, and ensure long-term alignment with their strategic goals. Success with AI isn’t just about innovation—it’s about continually refining the path to value.