AI’s role in helping the automotive sector adapt to increasing regionalization in 2025
Published April 28, 2025
- Automotive & Industry
- Data & AI
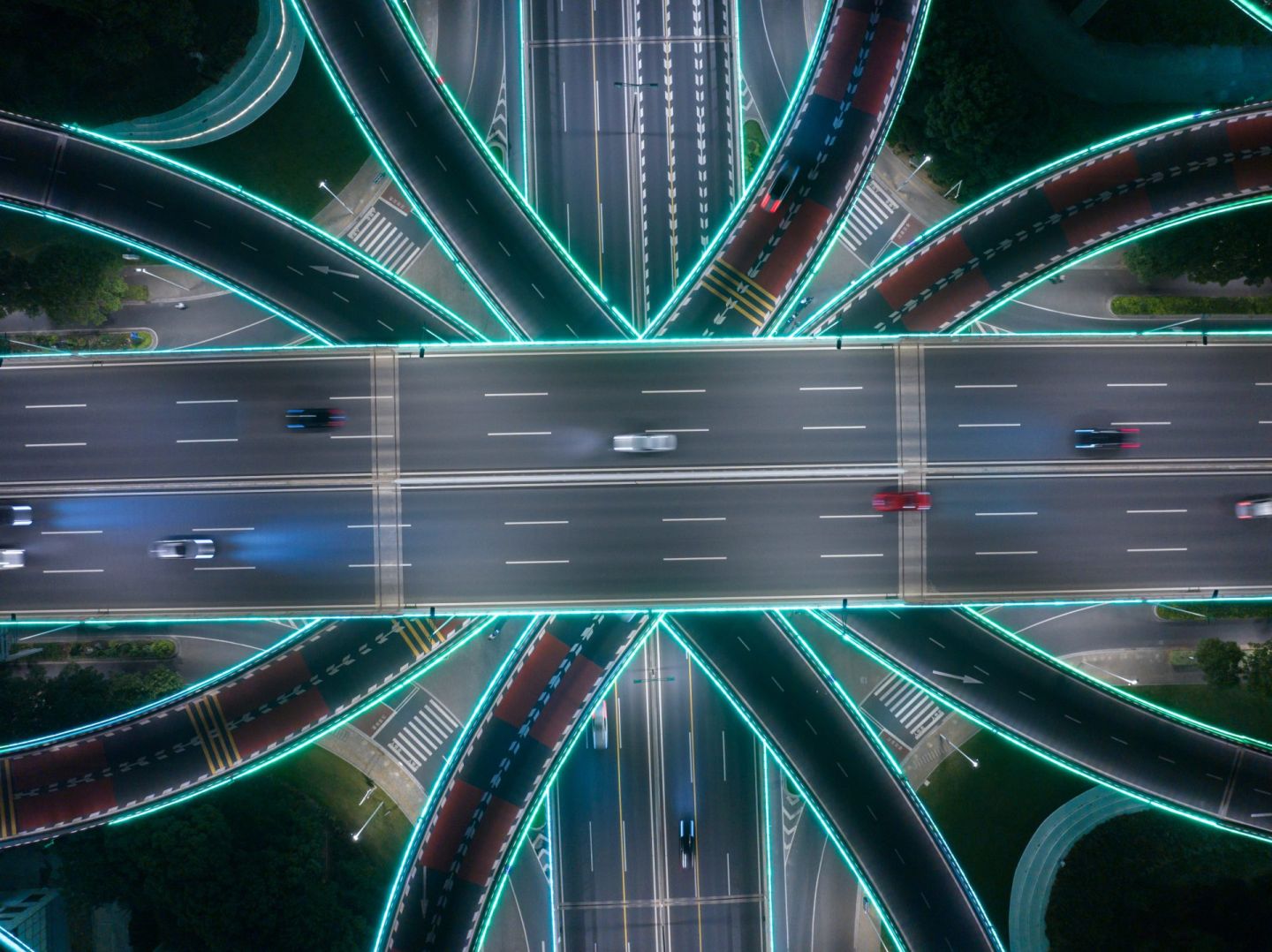
This article has primarly been published on La Tribune media.
Between 2015 and 2025, the global automotive industry saw modest annual growth of 0.3%, with sales rising from 88 to 91 million vehicles. This global stagnation masks divergent regional trends. In China, for example, sales of electric vehicles (EVs) have exploded, accounting for 25% of the market by 2025, up from just 5% in 2015. Meanwhile, Western markets have struggled to maintain their momentum, faced with complex regulatory and technological transitions.
As the industry enters a new phase, two structural forces are redefining the priorities of Western automakers: accelerated regionalization and the strategic adoption of artificial intelligence. These dynamics promise to profoundly transform the automotive value chain.
The irreversible trend towards regionalization
The globalization that dominated the automotive sector for decades is gradually giving way to regional strategies. Consumer preferences now differ considerably from region to region. In Europe, for example, small electric cars dominate, supported by government subsidies and strict regulations. Conversely, in North America, SUVs and pick-ups continue to account for an overwhelming share of sales, reaching over 70% by 2025. In Asia, demand is focusing more on compact, connected vehicles, in response to growing urbanization and the rise of a middle class.
Geopolitical tensions and protectionist policies also reinforce this trend. Faced with the uncertainties associated with tariffs and trade restrictions, automakers are now favoring local investments. Toyota, for example, has announced an $8 billion investment plan in the United States, aimed at boosting its electric vehicle production capacity in the region. At the same time, the pandemic and supply chain disruptions have revealed the fragility of global networks, prompting automakers to favor regional approaches to securing their operations.
Technologies that reconcile regional customization and economies of scale
Regionalization raises a major strategic challenge for automakers: how to reconcile their constant quest for economies of scale with increasingly specific local expectations? The use of global modular platforms is already a response to this problem. Volkswagen, for example, uses its MEB platform to produce models adapted to different regions, from the compact ID.3 in Europe to the SUV ID.4 in China and the USA. As Herbert Diess explained at the Group’s Annual Meeting in 2021, this approach should reduce unit costs by around 20%, while meeting the varied needs of consumers.
Digital tools are also transforming the way automakers personalize their offerings. Tesla, for example, enables its customers to configure their vehicles online with options specific to regional conditions. Consumers in Scandinavia, for example, can opt for batteries adapted to cold climates, while those in arid regions benefit from enhanced cooling systems. These innovations are not limited to production, but also extend to the customer experience, illustrating a fundamental shift in the relationship between brands and their consumers.
AI is profoundly altering automakers’ historic value chain
Artificial intelligence is becoming an indispensable tool for meeting the challenges posed by the regionalization of the automotive sector. It is transforming the entire automotive value chain, from supply management to product and service personalization. It enables us to meet the challenges of this regionalization, while at the same time improving operational efficiency, which is inevitable and expected globally.
In a context where automotive markets are fragmenting, automakers need to meet varied local expectations while maintaining global efficiency. And AI is the cornerstone of this dual success. It supports intelligent expansion strategies by enabling companies to meet the demands of personalization (products and services) and regionalization. This involves fine-tuned analysis of local data to identify opportunities and structure agile implementation models, such as joint ventures or partnerships. For example, Stellantis recently collaborated with Leapmotor to accelerate its presence in Asia, combining an advanced technological approach with a detailed understanding of the local market. Here, AI is used to optimize product configurations, anticipate the specific needs of the Chinese market, and structure a suitable distribution network, demonstrating how centralized intelligence can adapt to a variety of local contexts.
In supply chains, AI makes it possible to anticipate and react with agility to disruptions. Ford, for example, uses algorithms to reduce interruptions linked to semiconductor shortages by 25%, by identifying and redirecting critical flows in real time. This ability to anticipate goes hand in hand with inventory optimization, as demonstrated by BMW, which has managed to cut inventory costs by 18% while increasing the availability of critical parts in its European plants.
AI also plays a central role in sales personalization. General Motors uses customer data to fine-tune its sales proposals. In Asia, for example, where consumers prioritize connectivity, the integration of advanced and tailored infotainment systems is said to have led to a 12% increase in sales. Hyundai has also used this technology to design targeted marketing campaigns, increasing conversion rates by around 15% thanks to tailored offers such as extended warranties for electric batteries.
Last but not least, AI also makes it possible to take environmental requirements into account. A notable example is Tesla, which integrates adaptable manufacturing processes. In China, the company identified the interest, then designed specific models with customized features, such as HEPA filters to cope with air pollution. Meanwhile, in Europe, its offerings focus on energy efficiency improvements to meet consumers’ ecological expectations and local regulations.
Data reliability: a priority for transforming the value chain
While AI offers unique opportunities, its full effectiveness depends on the quality and reliability of data. Yet automakers face critical issues of data fragmentation and heterogeneity.
One of the main difficulties lies in the multiplication of data sources and data enrichment: vehicle sensors, customer interactions, multiple professions, third-party partners – all of which generate incomplete or inconsistent information. For example, data on the state of electric vehicle batteries can vary according to weather conditions or collection methods, making predictive analysis by AI algorithms difficult.
Similarly, data from third-party partners, such as assembly line parts suppliers, can suffer from a lack of standardization. Variations in the format or frequency of updates to critical component stock information then make it difficult to integrate into predictive supply management models, and limit manufacturers’ ability to anticipate and respond to supply chain disruptions. Without standardized, reliable data, algorithms produce erroneous predictions, compromising decision-making.
What’s more, data interoperability between different professions at automakers, or now their intelligent agents, remains a major challenge. Take the example of technical data issued by the design-engineering department: if its semantic definition is not harmonized, it could be difficult for the supply chain team to exploit. This lack of coordination slows down the potential efficiency gains offered by AI, whether in terms of optimizing logistics flows or reducing production lead times.
To overcome these obstacles, it is essential to decompartmentalize data and make it more reliable. Unified, interoperable platforms make it possible to aggregate data from different sources, while reducing duplication and establishing robust standards to guarantee data quality. Some manufacturers, such as Stellantis, exemplify pieces of this approach: by centralizing data from its multiple brands on a single platform, the manufacturer has increased the accuracy of its operational forecasts and reduced inconsistencies.
This transformation benefits not only internal management, but also the use of data by so-called intelligent agents. The latter can then offer precise, tailored recommendations, whether to personalize commercial offers, plan complex logistics operations or predict the future needs of a regional market. In this way, data reliability becomes a sine qua non condition for exploiting the full potential of AI and transforming the automotive value chain.
Towards an agile future
The automotive industry is at a crossroads. Market fragmentation and AI represent major currents for reinventing business models and meeting the expectations of an increasingly diverse customer base. Manufacturers who invest intelligently in their data, and robust, unified data management systems, while adapting their offerings to regional specificities, will be able to capture the value created by these transformations. Regionalization and artificial intelligence are not simply passing trends, but transformative forces.
Companies that know how to combine these dynamics will have a substancial competitive advantage in 2025 and beyond.
Author
-
Mathieu Sabarly
Associate Partner – France, Paris
Wavestone
LinkedIn